Three protein design tools to use in 2023
- Ranomics
- May 31, 2023
- 2 min read
Computational protein design is a rapidly evolving field that aims to create new proteins with desired structures and functions. Computational tools play a crucial role in this process, as they enable the exploration of vast sequence spaces and the prediction of protein stability and interactions. In this blog post, we will introduce some of the computational tools that are widely used for aiding protein design and discuss their advantages and limitations.
One of the most popular computational tools for protein design is Rosetta, a software suite that includes algorithms for modeling and analyzing protein structures. Rosetta can perform various tasks such as de novo protein design, enzyme design, ligand docking, and structure prediction of biological macromolecules and macromolecular complexes. Rosetta uses a physics-based scoring function that incorporates properties such as van der Waals, electrostatics, solvation, and entropy to evaluate the energy of a protein conformation. Rosetta also uses knowledge-based terms such as rotamer libraries and backbone dihedral preferences to guide the sampling of protein conformations. Rosetta has been successfully applied to design novel folds, enzymes, vaccines, antibodies, protein assemblies, ligand-binding proteins, and membrane proteins [1].
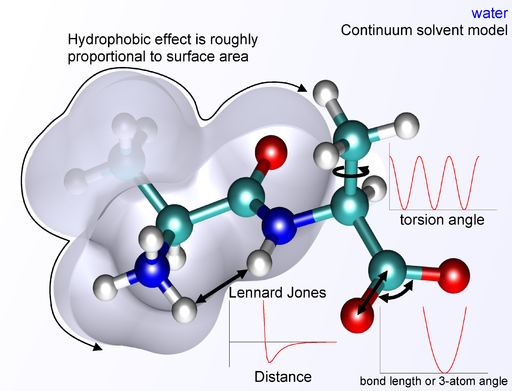
Another computational tool that has recently emerged for protein design is RoseTTAFold, a deep learning neural network that can quickly and accurately predict protein structures from amino acid sequences alone. RoseTTAFold is a three-track neural network that simultaneously considers patterns in protein sequences, how amino acids interact with each other, and possible three-dimensional structures. RoseTTAFold has been used to compute hundreds of new protein structures, including many poorly understood proteins from the human genome and proteins associated with human health and disease [2]. RoseTTAFold can also be used to build models of complex biological assemblies in a fraction of the time previously required [3].
A third computational tool that is gaining attention for protein design is RF Diffusion, a generative model that can create new proteins with desired properties. RF Diffusion is a guided diffusion model that specializes in adding and removing noise from protein sequences. RF Diffusion can outperform existing protein design methods across a broad range of problems and has been used to generate ultra-high affinity binders and novel symmetric assemblies that have been experimentally validated [4]. RF Diffusion can generate diverse and high-quality protein designs with minimal experimental testing.
These are just some examples of the computational tools that are available for aiding protein design. Computational protein design is a powerful tool to engineer new functional capabilities in proteins and to expand the capabilities of synthetic biology. However, computational tools are not perfect and still face challenges such as accuracy, efficiency, scalability, and generalizability. Therefore, computational tools should be used in combination with experimental validation and optimization to achieve the best results.
References:
[1] Koehler Leman J., Weitzner B.D., Lewis S.M., et al. Macromolecular modeling and design in Rosetta: recent methods and frameworks. Nat Methods 17 , 665–680 (2020).
[2] Baek M., DiMaio F., Anishchenko I., et al. Accurate prediction of protein structures and interactions using a three-track neural network. Science 373 , 871–876 (2021).
[3] Anishchenko I., Ovchinnikov S., Kamisetty H., et al. De novo protein structure prediction by deep learning without co-evolutionary information. bioRxiv (2021).
[4] Yang K., Wu Z., Bedbrook C.N., et al. Learned Protein Embeddings for Machine Learning. bioRxiv (2020).
Kommentare